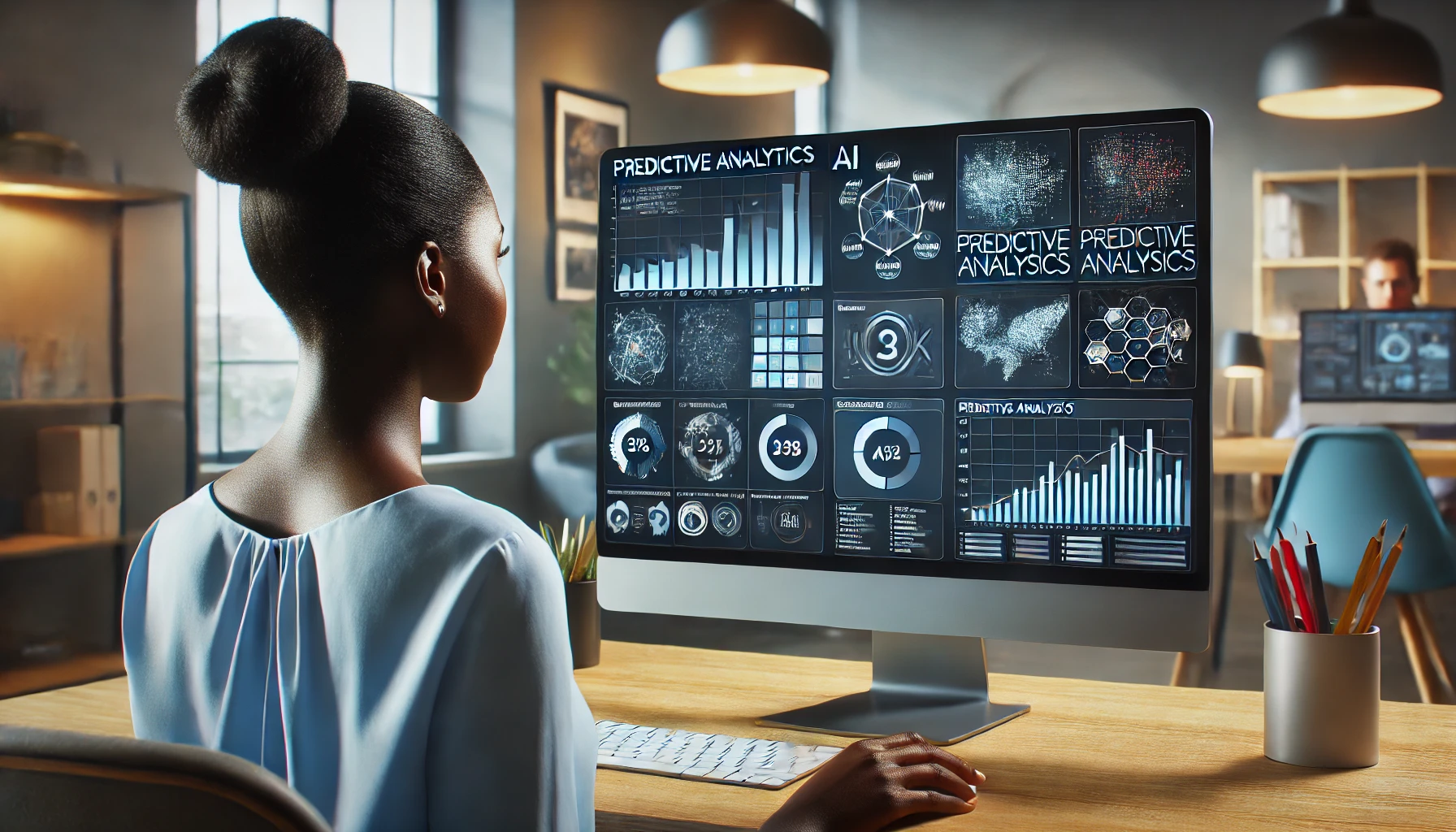
How to Best Use AI for Predictive Analytics
One of the most significant challenges is predicting future trends and behaviors to make informed decisions. Enter AI for predictive analytics, a powerful tool that transforms raw data into actionable insights. This article will explore how businesses can best leverage AI for predictive analytics, providing relevant information, statistics, and recent news on the subject.
What is Predictive Analytics?
Predictive analytics is a powerful methodology that involves analyzing historical data to make informed predictions about future events. This process relies on statistical algorithms and machine learning techniques to uncover patterns and relationships within the data. By examining past trends and behaviors, predictive analytics can estimate the probability of various outcomes, helping businesses make data-driven decisions. For example, a retailer might use predictive analytics to forecast inventory needs based on previous sales data, while a healthcare provider could predict patient admission rates by analyzing historical health records. The core strength of predictive analytics lies in its ability to transform raw data into actionable insights, enabling organizations to anticipate changes and adapt strategies proactively.
AI significantly enhances predictive analytics by automating complex calculations and sophisticated pattern recognition. Traditional predictive models often require extensive manual input and expert knowledge to fine-tune, which can be time-consuming and prone to human error. AI addresses these challenges by leveraging advanced algorithms that continuously learn and improve from the data. This automation not only increases the speed and efficiency of the analytics process but also boosts its accuracy. AI can handle vast datasets with multiple variables, identifying subtle correlations that might be overlooked by human analysts. As a result, businesses can rely on AI-enhanced predictive analytics to make more precise forecasts, optimize operations, and gain a competitive edge in their respective markets.
Automation and AI in Predictive Analytics
Automation in predictive analytics enables businesses to process vast amounts of data quickly and accurately. According to a report by Gartner, the worldwide end-user spending on robotic process automation (RPA) is projected to reach $2.9 billion in 2022, reflecting the growing importance of automation in business processes.
Moreover, research by McKinsey suggests that AI can enhance predictive analytics by providing deeper insights through advanced data processing and machine learning algorithms. For instance, predictive maintenance in manufacturing can reduce downtime by up to 50% and increase machine life by 20-40%.
Key Benefits of AI for Predictive Analytics
Leveraging AI for predictive analytics offers a multitude of benefits that can significantly enhance business operations and strategic planning. One of the most impactful advantages is improved decision-making. AI-driven predictive analytics provides businesses with highly accurate forecasts by analyzing vast amounts of data and identifying patterns that human analysts might miss. This allows companies to anticipate market trends, understand customer behavior, and assess potential risks more effectively. With these insights, businesses can make better-informed strategic decisions, such as optimizing product launches, targeting marketing efforts, and allocating resources more efficiently.
Increased efficiency is another key benefit of incorporating AI into predictive analytics. Traditional data analysis methods often require significant manual effort and time, which can be resource-intensive and slow. AI automates these processes, enabling the rapid processing of complex datasets with minimal human intervention. This not only saves time but also frees up valuable resources, allowing businesses to focus on their core activities and strategic initiatives. Additionally, AI’s ability to handle large-scale data analysis quickly ensures that businesses can respond to changes in the market environment promptly, maintaining a competitive edge.
Enhanced AI customer experience is also a significant benefit of using AI-driven predictive analytics. By accurately predicting customer needs and preferences, businesses can personalize their offerings and deliver tailored experiences that meet individual customer expectations. This leads to higher customer satisfaction and loyalty, as customers feel valued and understood. For instance, a retailer might use predictive analytics to recommend products based on a customer's previous purchases, while a service provider could tailor their communication strategies to align with customer preferences.
Cost reduction is another substantial advantage offered by AI-enhanced predictive analytics. By identifying inefficiencies within operations and highlighting areas for improvement, businesses can streamline processes and reduce waste. For example, predictive maintenance can forecast when equipment is likely to fail, allowing for timely interventions that prevent costly downtime. Similarly, optimized supply chain management driven by predictive analytics can reduce excess inventory and minimize storage costs. Ultimately, these efficiencies translate into significant cost savings, improving the overall financial health of the business.
Here are the AI regulations you should know.
Recent Developments in AI and Predictive Analytics
Recent developments in AI and predictive analytics have seen significant advancements, with major technology companies like IBM leading the charge. IBM recently introduced a cutting-edge AI-driven predictive analytics solution aimed at helping businesses forecast market trends and optimize their operations. This innovative tool utilizes sophisticated machine learning algorithms to process and analyze historical data, providing highly accurate predictions about future outcomes. By automating the data analysis process, IBM's solution enables businesses to gain deep insights into market dynamics, customer behavior, and operational efficiency, thereby facilitating more informed decision-making.
The introduction of IBM's predictive analytics solution marks a significant milestone in the broader trend of integrating AI into business analytics. As the volume of data generated by businesses continues to grow exponentially, traditional data analysis methods struggle to keep pace. AI-driven solutions like IBM's not only manage large datasets more efficiently but also enhance the accuracy and reliability of the insights derived. This integration of AI allows businesses to move beyond descriptive analytics, which focuses on past performance, to predictive analytics, which provides a forward-looking perspective. Consequently, companies can proactively address potential challenges and seize opportunities, ultimately driving greater business value.
IBM's latest offering is part of a broader wave of advancements in AI and predictive analytics, reflecting the increasing importance of these technologies in the modern business landscape. The ability to predict future trends with high accuracy is becoming a crucial competitive advantage, as businesses that can anticipate market changes and adapt accordingly are better positioned to thrive. This trend is evident across various industries, from retail and finance to healthcare and manufacturing, where AI-driven predictive analytics is being used to enhance customer experience, optimize supply chains, improve operational efficiency, and reduce costs. As more companies adopt these advanced analytics tools, the role of AI in shaping strategic business decisions is set to become even more prominent.
Read more: AI tools in digital marketing
Practical Steps for Implementing AI in Predictive Analytics
- Data Collection and Preparation: Gather relevant data from various sources. Ensure the data is clean, accurate, and formatted for analysis.
- Choosing the Right Tools: Select AI-powered predictive analytics tools that suit your business needs. Popular options include IBM Watson, Google Cloud AI, and Microsoft Azure Machine Learning.
- Model Building and Training: Develop machine learning models tailored to your specific use case. Train the models using historical data to ensure accuracy.
- Integration and Deployment: Integrate the predictive models into your business processes. Monitor performance and make necessary adjustments to improve accuracy.
- Continuous Improvement: Regularly update the models with new data and refine them to adapt to changing business conditions.
Conclusion
AI for predictive analytics offers businesses a powerful tool to anticipate future trends, optimize operations, and enhance decision-making. By leveraging automation, companies can unlock valuable insights and stay competitive in an ever-evolving market. As recent advancements by industry leaders like IBM demonstrate, the integration of AI into predictive analytics is not just a trend but a necessity for forward-thinking businesses.
Embrace the power of AI for predictive analytics to transform your business and gain a strategic edge in today's data-driven world.